Understanding the Need for AI in Media
Before we dive into the various AI applications being used in media, let's take a closer look at why there is a clear need for AI in this space.
Over the years, media consumption has changed dramatically. People now expect fast, engaging content. Short-form videos, live streams, and constantly updated feeds are the new normal. Platforms like TikTok, YouTube Shorts, and Instagram Reels have reshaped how and what people watch. As a result, the volume and speed of content creation have skyrocketed.
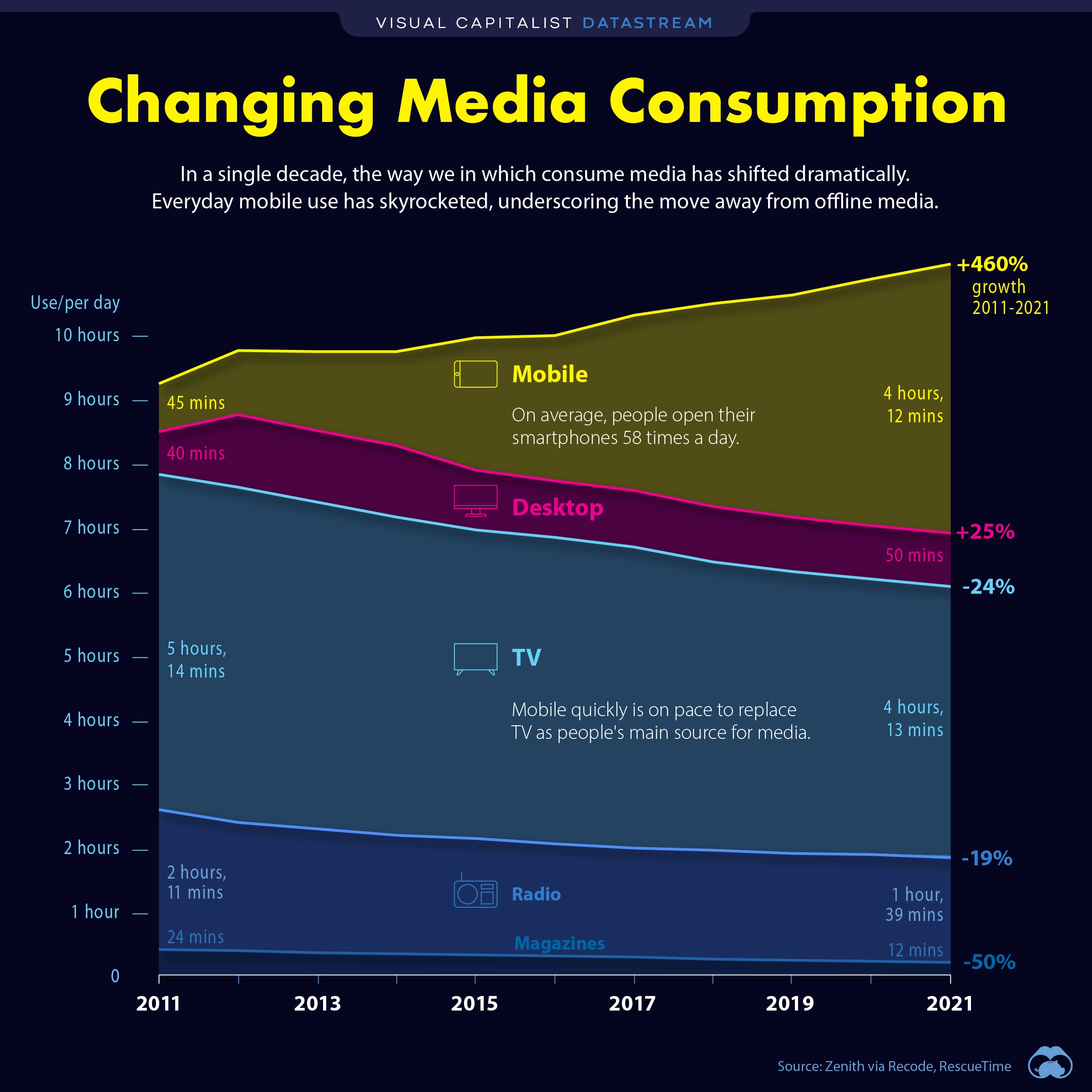
How Media Consumption has Changed Over the Years (Source)
With so much content being created and shared every second, there’s a growing need for real-time content handling. Media companies can no longer rely on manual processes to sort, tag, summarize, and recommend content.
It would be like trying to organize a library where thousands of new books appear on the shelves every minute, without a catalog system or any help. We’d quickly fall behind, and important content would get lost in the chaos.
AI makes it possible to create faster, more scalable, and more accurate workflows. Tasks like video summarization, image tagging, caption generation, and content recommendation can now be automated or greatly enhanced with AI. The key advantage is that AI solutions in media can save time and increase efficiency.
This shift benefits a wide range of industries. News outlets delivering up-to-the-minute coverage, streaming services personalizing user experiences, and social media platforms managing massive volumes of content can all use AI innovations.
Image Summarization Enabled by AI
Let’s say you’re part of a news team covering a major event. Hundreds of photos come in from photographers on the ground, and you need to find the best ones to publish quickly. Going through each image manually would take hours.
However, image summarization can be used to automatically scan and analyze each photo, identify what’s in it, like people, objects, or scenes, and generate short descriptions or tags. This makes it much easier to sort through the images, search for what you need, and select the most relevant ones quickly.
Using AI and computer vision, image summarization helps systems see and understand the content in images. It can recognize objects like faces, logos, landmarks, or text, and even group similar images together. This kind of automation saves time, reduces human error, and helps teams work more efficiently.
Beyond newsrooms, image summarization can be used in many other industries, like e-commerce for product tagging, social media platforms for organizing user photos, and marketing teams managing large libraries of creative assets. It’s a simple but reliable way to handle large volumes of visual content without getting overwhelmed.
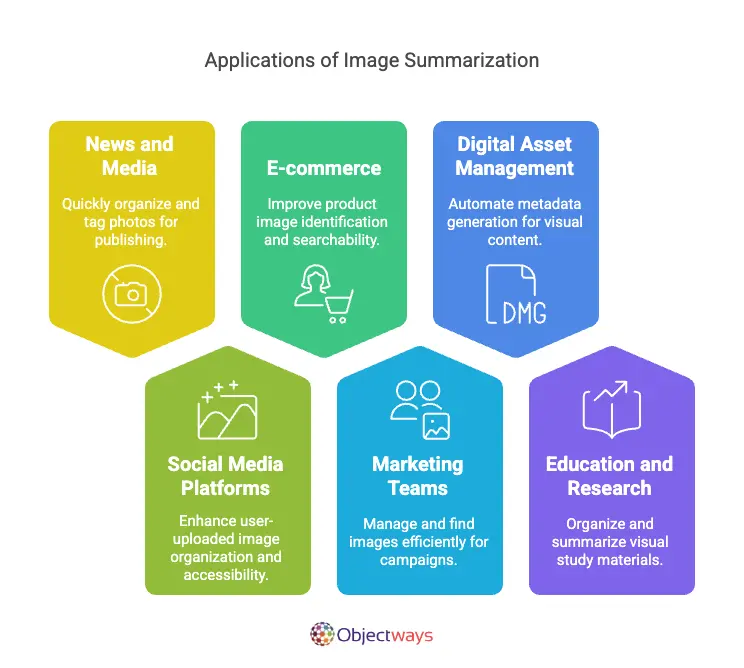
Applications of Image Summarization
Exploring AI Video Summarization
Similar to image summarization, AI video summarization helps make sense of large amounts of video content by automatically picking out the most important parts. Instead of watching an entire video, viewers can get a quick summary or highlights.
These AI in media systems use a mix of tools, like computer vision and natural language processing, to understand what's happening in the video, from spoken words to scene changes and visual details. It can either pull out key clips (extractive) or create a shorter version in its own words (abstractive).
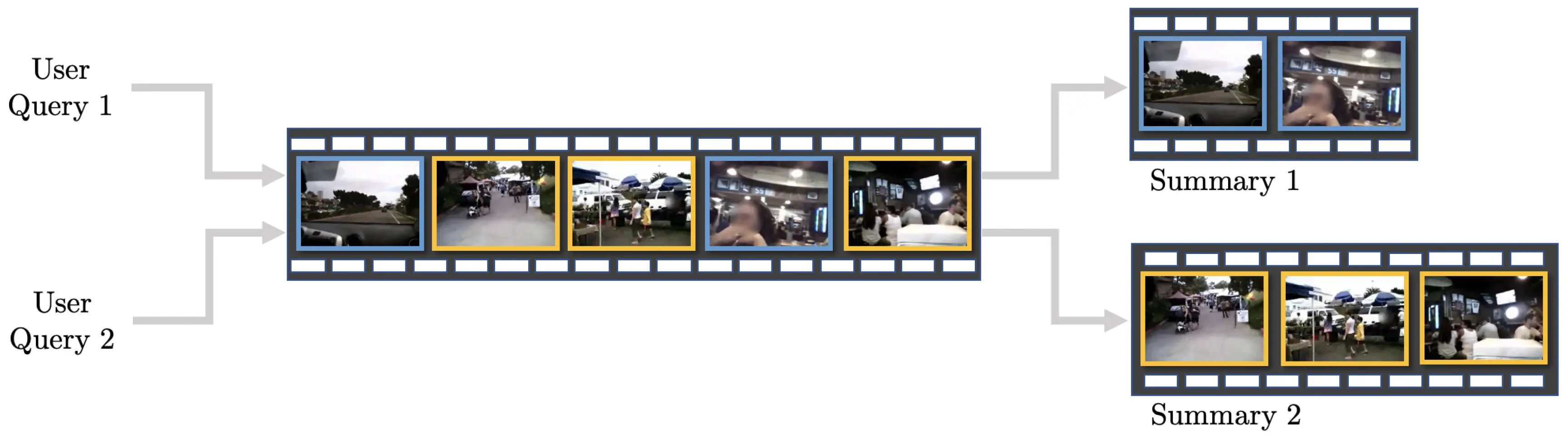
Creating Video Summaries Based on User Queries. (Source)
This technology is already being used in plenty of impactful applications. Here’s a glimpse at some of these applications:
- Streaming Platforms: Platforms like Netflix or YouTube can use AI to automatically generate previews or summaries of shows and movies, giving viewers a quick sense of the content.
- Social Media: Long-form videos can be automatically clipped into shorter, shareable content for platforms like Instagram Reels, TikTok, or YouTube Shorts.
- Video Archives and Search: With AI-generated summaries, large video libraries become easier to search and navigate, helping users find the exact moment or topic they need.
Making Content Accessible Through Live Captioning
Media is a huge part of our everyday lives, and that’s what makes it so crucial for content to be accessible to everyone. An interesting technology that is making content more accessible is live captioning. Live captioning is the real-time display of spoken words as text on a screen during live events, broadcasts, or video streams.
It is especially helpful for people who are deaf or hard of hearing, but they’re useful for many other situations as well. Maybe someone is watching without sound, in a noisy place, or trying to follow along in a language they don’t speak fluently.
AI can make live captioning much faster and easier to deliver. With tools like automatic speech recognition (ASR) and natural language processing (NLP), AI models can turn spoken words into text almost instantly. These models are also getting better at understanding different voices, accents, and background noise.
A good example of live captioning in action is Apple’s support for it on the iPhone, Mac, and Apple Watch. This Apple feature can be used for real-time captions for nearly any audio, FaceTime calls, videos, podcasts, or in-person conversations, right on the device. For FaceTime, it even shows a scrolling transcript that identifies who’s speaking.
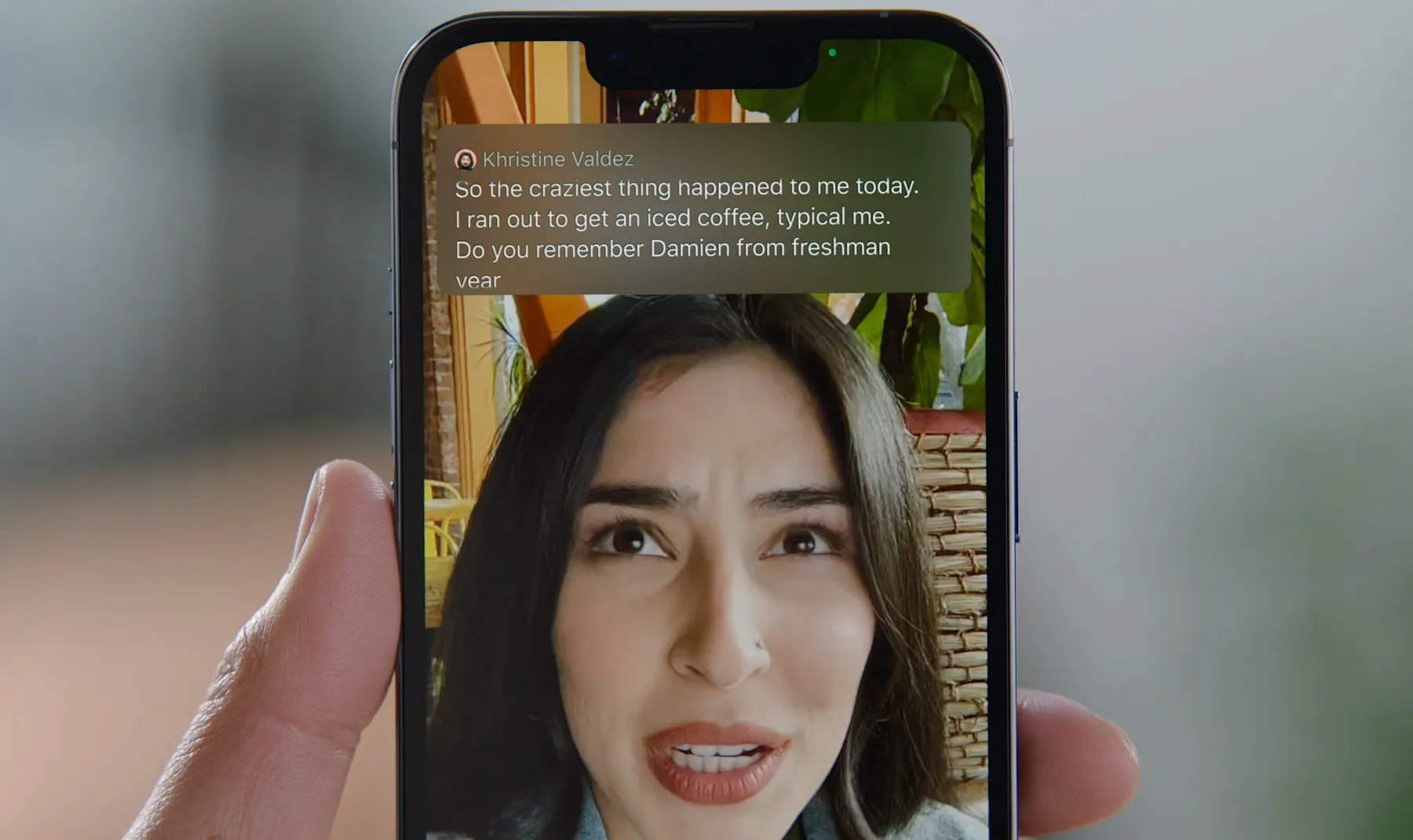
FaceTime supports live captioning. (Source)
AI in Content Recommendation Systems
When you’re scrolling through your favorite streaming platform like Netflix or browsing an online store, it often feels like the system already knows what you’ll want next. That’s because behind the scenes, AI-driven content recommendation systems are working to personalize your experience. They analyze your behavior - what you’ve watched, clicked on, searched for, or ignored - and use that information to suggest content or products that match your interests.
A particularly fascinating example from Netflix is that even the poster art for a movie or show is personalized based on your preferences. For instance, if you usually watch romantic comedies, the thumbnail might highlight the romantic storyline, while someone else might see a more action-focused or comedic version of the same title.
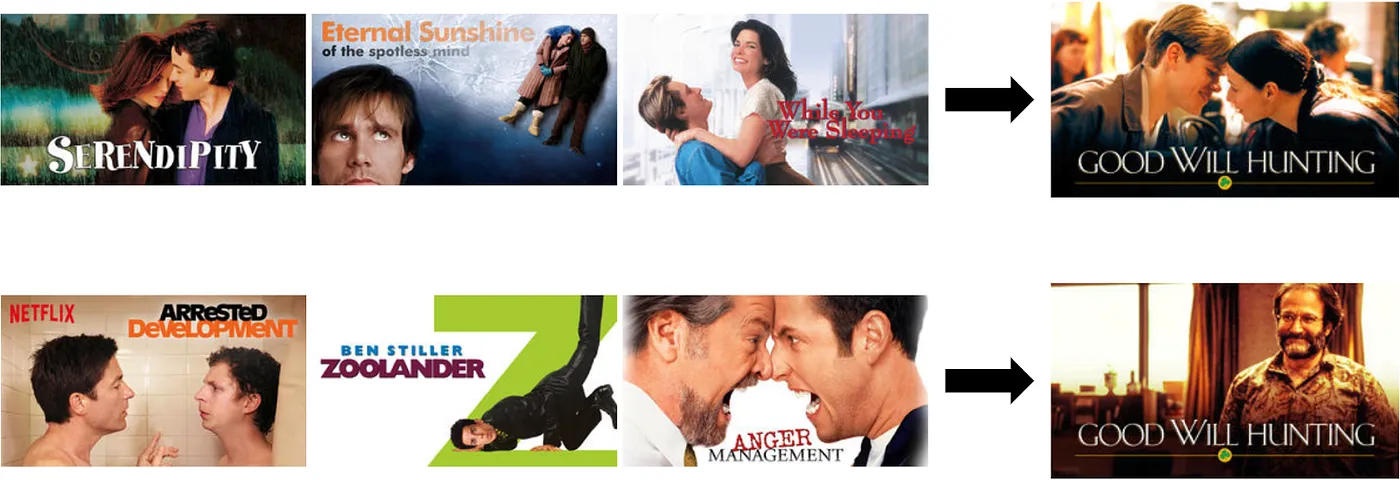
Netflix personalizes movie posters. (Source)
How Do Content Recommendation Systems Work?
Content recommendation systems use a few different methods to make accurate recommendations. One is collaborative filtering, which finds users with similar tastes and suggests what they liked. You can compare it to asking a group of friends who share your interests what movies or books they enjoyed - chances are, you’ll probably like them too. These systems generally compare patterns across millions of users to make smart suggestions based on shared preferences.
Another technique is content-based filtering. It looks at the details of the content you've interacted with and finds similar items. Think of it like finding more songs that sound like your favorite track or shows that share the same genre, tone, or actors - it’s all about matching the qualities of things you already enjoy.
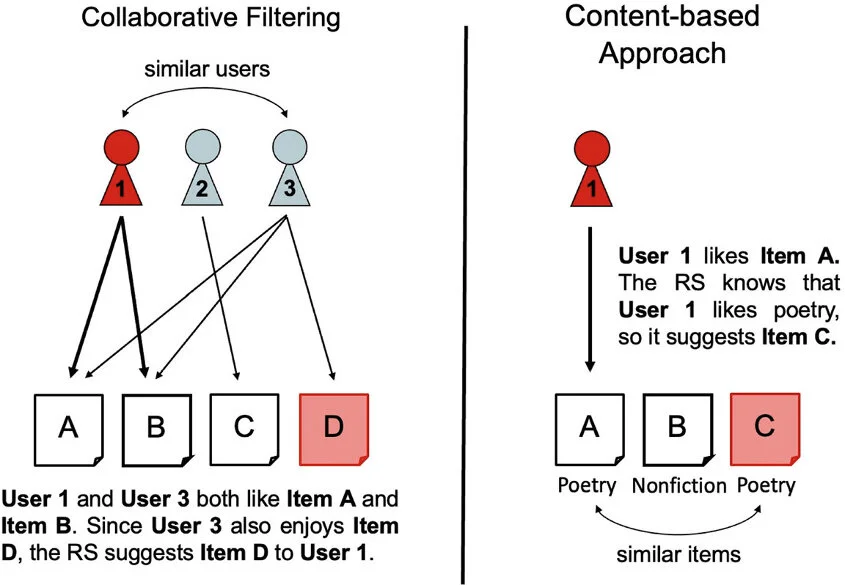
Collaborative Filtering Vs. Content-Based Filtering (Source)
Many platforms use a hybrid approach, combining both methods for even better results. The AI models integrated into these systems process huge amounts of data in real time, learning from your activity and constantly adjusting their suggestions to be more relevant. This technology powers recommendations on platforms like Netflix, Amazon, Spotify, YouTube, and even news apps - helping you discover new things quickly and easily.
Challenges Related to Implementing AI in Media
While AI is changing how media is created, shared, and experienced, putting it into practice isn’t always simple. Behind tools like video summarization, live captioning, and content recommendation systems, there are technical limitations that need to be considered.
Here are some of the common challenges related to implementing AI in media:
- Data Quality and Bias: AI systems learn from large amounts of data, but if that data is biased or incomplete, the results can be inaccurate or unfair.
- Accuracy and Context: AI models don’t always understand the whole meaning behind what’s being said or shown, especially when it comes to slang, humor, or cultural context.
- Lack of Transparency: It’s often hard to know exactly how AI systems make decisions, which can make it difficult to trust or fine-tune the results.
- Privacy and Data Protection: AI solutions often rely on user data, so it’s important to handle that information carefully and follow privacy regulations.
That’s why having the right AI expertise on your team really matters. If you’re looking for support to bring AI into your media projects, Objectways can help. We specialize in high-quality data labeling and custom AI solutions that make it easier to build smarter, more effective tools.
The Road Ahead with AI in Media
The future of AI in media is full of exciting possibilities. From making content easier to discover and understand to improving accessibility and personalizing the user experience, AI is quickly becoming a core part of how media is created and consumed.
While there are still challenges to overcome, the potential benefits are also substantial. With the right tools and expertise, media companies can use AI to work smarter, move faster, and connect better with their audiences.
If you're ready to explore how AI can improve your media workflows, Objectways is here to help. Contact us today to learn how our data labeling services and custom AI solutions can support your next project.
Frequently Asked Questions
- How is AI used in the media?
- AI helps automate content creation, tagging, summarization, recommendation, and accessibility. It improves workflows, personalizes user experiences, and makes it easier to manage large volumes of media content.
- What is a video summarization?
- Video summarization is the process of using AI to automatically generate shorter versions of videos by highlighting key moments, scenes, or information, making content easier to browse and consume.
- What is the meaning of live captioning?
- Live captioning is the real-time display of spoken words as on-screen text during live events, broadcasts, or video calls, helping improve accessibility for all viewers, especially the hearing-impaired.
- What is an example of a content recommendation?
- When Netflix suggests a movie based on what you’ve watched before, that’s a content recommendation. AI analyzes your preferences and shows similar content that you might enjoy.